Analytics in Recruitment: A Comprehensive Guide to Data-Driven Talent Acquisition
Understanding Analytics in Modern Recruitment
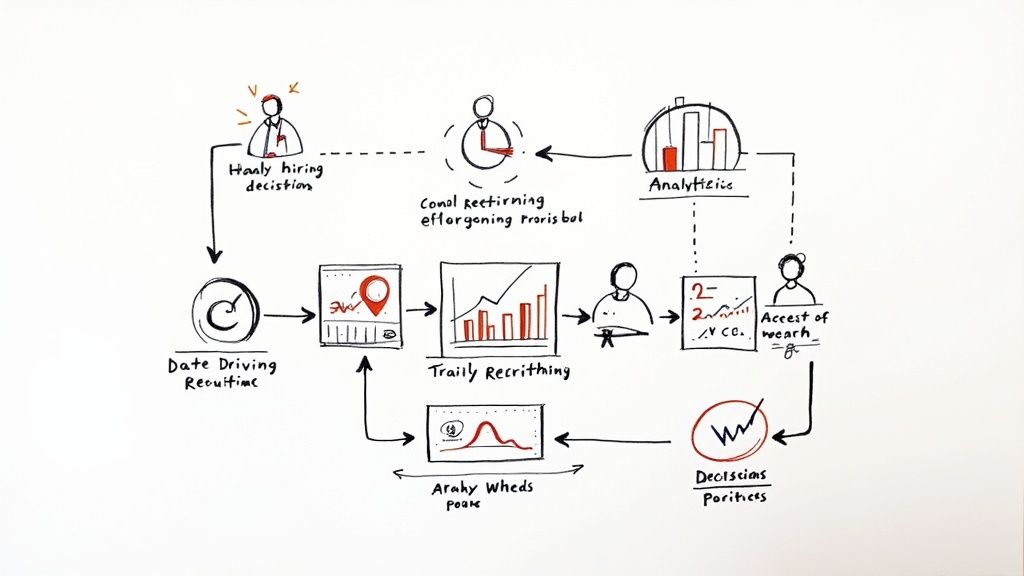
Successful recruitment today relies on concrete data and insights rather than gut feel alone. Organizations that analyze key metrics make better hiring decisions and build stronger teams. The most impactful metrics include time-to-hire, cost-per-hire, source effectiveness, and quality of hire. Getting familiar with these numbers is essential for creating an effective recruitment strategy.
Key Metrics in Recruitment Analytics
Several numbers help measure recruitment success. For example, time-to-hire shows exactly how long it takes to fill open roles, making it easier to spot slowdowns in the process. Similarly, cost-per-hire helps teams track spending on each new employee and calculate returns on their recruitment investments.
- Time-to-Hire: The total days from job posting to accepted offer
- Cost-per-Hire: All expenses related to filling a position
- Source of Hire: Which channels bring in the best candidates
- Offer Acceptance Rate: Percentage of candidates who say yes
- Quality of Hire: How well new employees perform in their roles
Today's recruitment data goes far beyond just tracking basic numbers.
The Power of Predictive Analytics
Predictive analytics helps companies make smarter decisions about future hiring needs. By looking at past data patterns, teams can forecast which candidates are most likely to succeed in specific roles. Tools like regression analysis and decision trees help process candidate skills, experience, and behavior to spot top talent. These data-driven methods improve hiring accuracy and quality.
Building a Data-Driven Recruitment Culture
Using analytics effectively requires more than just collecting numbers - it needs a data-focused mindset throughout the hiring team. This means training recruiters and managers to understand data and apply insights at every stage, from sourcing to onboarding. When teams consistently use data to guide decisions, they build stronger talent pipelines and make better hires. A data-driven approach gives organizations an edge in attracting and keeping great employees.
Maximizing Recruitment ROI Through Data Intelligence
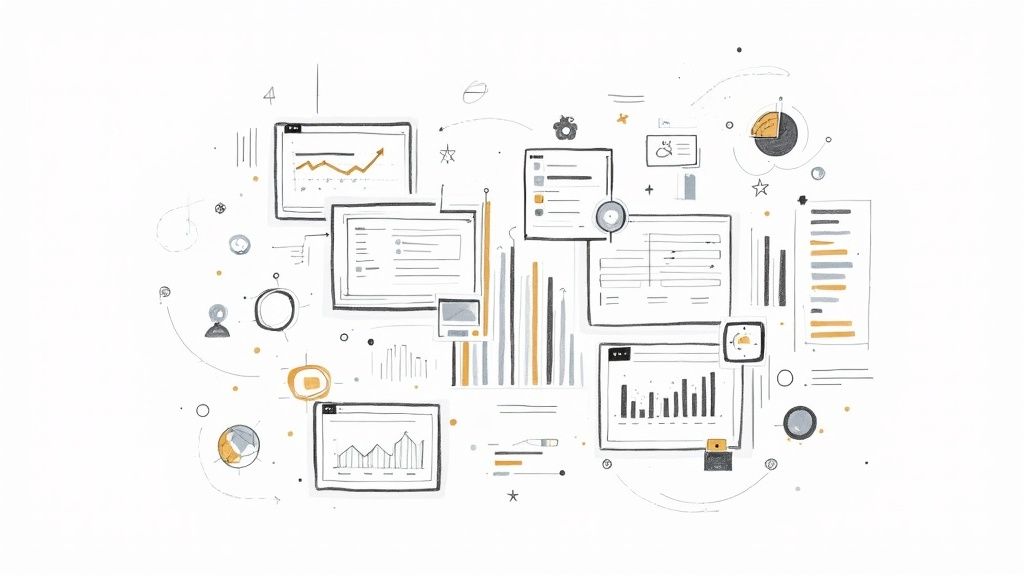
Companies are using data and analytics to measure and improve their return on investment in recruiting. Smart organizations now analyze every step of talent acquisition - from sourcing candidates to making job offers. This detailed analysis helps identify opportunities for improvement and shows the real value of recruitment efforts.
Calculating True Cost-Per-Quality-Hire
Going beyond basic cost metrics, cost-per-quality-hire looks at how new employees perform and how long they stay. A lower initial hiring cost might seem good, but if those hires underperform or leave quickly, the real cost is much higher. Companies use data on employee performance, retention, and other key factors to understand the actual cost of each hire. This helps make better hiring decisions that lead to improved ROI.
Optimizing Budget Allocation Based on Channel Performance
Data helps companies put their recruiting budgets where they get the best results. By tracking how different sources like job boards, social media, and employee referrals perform, recruiters can focus spending on channels that deliver quality candidates cost-effectively. Teams can also fine-tune their approach on each platform, like adjusting job descriptions or targeting specific candidate groups.
Identifying and Addressing Hidden Cost Drivers
Many costs in recruiting aren't obvious at first glance. These can include long hiring timelines, inefficient processes, or poor onboarding. Data analysis reveals these hidden issues by showing bottlenecks and problems in the hiring process. For example, looking at onboarding data might show opportunities to use more cost-effective tools and methods. Learn more about reducing recruitment costs through data-driven strategies here. Finding and fixing these hidden costs significantly improves overall recruitment ROI.
Demonstrating Recruitment ROI to Stakeholders
Data helps recruitment teams show their value clearly to company leaders. By sharing concrete metrics on hiring costs, time-to-fill positions, and candidate quality, recruiters prove how their work helps the company's bottom line. This data-based approach helps justify spending on recruitment tools and positions the recruiting team as key partners in achieving business goals. It also gives them more influence to improve hiring practices.
Creating Exceptional Candidate Experiences Through Data

A positive candidate experience is essential for successful hiring in the modern job market. Companies must actively engage candidates throughout the recruitment process, providing clear communication and smooth workflows. Analytics plays a key role by helping recruiters understand and improve each touchpoint in the candidate journey.
Using Data to Personalize the Candidate Journey
Analytics enables recruiters to create customized experiences for different candidate segments. By analyzing data, recruiters can determine which communication channels work best for specific groups and adapt their outreach accordingly. They can also identify candidates' key interests and priorities, allowing for more relevant and engaging job opportunity discussions.
Identifying and Eliminating Bottlenecks
By tracking metrics like time-to-hire and application completion rates, recruiters can spot where candidates encounter friction or delays in the hiring process. For example, if many candidates abandon applications midway, this may signal that the form is too complex or confusing. Early detection of these issues allows teams to make improvements before they impact hiring results.
Building a Strong Employer Brand Through Data
Happy candidates boost your employer brand by sharing positive experiences, even if they don't join your company. On the flip side, negative experiences can quickly damage your reputation. Analytics helps teams deliver better experiences by measuring key hiring metrics and making data-driven improvements. Learn more about optimizing your hiring process with analytics here.
Measuring the Impact of Candidate Experience Initiatives
Teams should track metrics like candidate feedback scores and offer acceptance rates to assess their candidate experience efforts. This data shows what's working well and where there's room for improvement. Regular measurement and optimization help build stronger talent pipelines and better hiring outcomes over time.
Building Predictive Models That Actually Work

Moving beyond basic metrics tracking, predictive modeling helps organizations forecast future hiring needs and make data-driven decisions. Success depends on carefully selecting the right data points, tools, and processes.
Identifying Key Predictive Factors
The foundation of effective predictive modeling is understanding which specific data points correlate with successful hires at your company. For sales roles, past sales performance data often proves valuable. For technical positions like software engineering, coding assessment scores may matter more. The key is analyzing your historical hiring data to find clear connections between candidate attributes and on-the-job success.
Selecting the Right Tools and Technologies
Companies have many options for predictive modeling tools - from basic spreadsheet software to specialized AI platforms like Recrew. Your choice depends on your model complexity and available resources. Some organizations do well with simpler tools, while others need robust solutions for large datasets. Recrew helps by automatically parsing resumes into structured data, giving you clean information for modeling.
Building and Refining Your Predictive Models
Creating effective models requires testing and refinement over time. Start by forming a hypothesis about success factors, test it against past data, then adjust based on results. Like athletic training, modeling demands ongoing practice to reach peak performance. Regular evaluation helps ensure models stay accurate and relevant as your hiring needs evolve.
Combining Human Expertise with Predictive Analytics
While data models provide valuable insights, they work best alongside human judgment. Think of it as a partnership - models offer recommendations while recruiters apply their expertise to evaluate candidates holistically. This balanced approach leads to better hiring decisions by combining data with human understanding. Regular collaboration between recruiters and analysts also helps identify and remove potential biases, improving model accuracy over time.
Measuring What Matters: Essential Recruitment Metrics
Good recruitment requires solid data, not just instinct. By focusing on the right performance indicators and metrics, teams can gain real insights into what's working and what isn't in their hiring processes. Making decisions based on data, rather than gut feelings, leads to better hires and a more efficient talent acquisition approach.
Key Recruitment Metrics and Their Impact
The most important metrics paint a clear picture of how well your recruiting is working. Time-to-hire shows how long it takes to fill roles and helps identify slowdowns in the process. When you reduce time-to-hire, you typically save money and create a better experience for candidates. Cost-per-hire helps track spending and shows the return on your recruiting investments. Taking it a step further, cost-per-quality-hire factors in how well new employees perform and how long they stay - giving you insight into the true value of each hire.
- Time-to-Hire: Measures the time taken to fill a position.
- Cost-per-Hire: Calculates the total cost of hiring a new employee.
- Source of Hire: Identifies the most effective recruitment channels.
- Offer Acceptance Rate: Tracks the percentage of accepted job offers.
- Quality of Hire: Assesses the performance and contribution of new hires.
Building Actionable Dashboards
To make good use of your data, create simple dashboards that show key metrics clearly. Track things like application completion rates to see where candidates might be dropping off in your process. You might find you need to simplify your application forms or make them work better on mobile devices. Watch your funnel conversion rates to spot where candidates tend to exit the hiring process.
Setting Realistic Benchmarks
Compare your metrics to industry standards to understand how you stack up. This helps identify areas where you're doing well and where you need work. For example, if your time-to-hire is much longer than average, you likely need to speed up your process. Low offer acceptance rates could mean your pay packages or employer brand need attention.
Reporting and Continuous Improvement
Regular reports help keep everyone informed about recruiting performance and show the value of your work. Update your approach based on what the data tells you - try new tools, adjust how you find candidates, or improve the candidate experience. Tools like Recrew, with its 99.9% accuracy in parsing resumes, can handle the data work so you can focus on making good decisions. When you consistently track and act on key metrics, you build a stronger hiring process that brings in great talent.
Emerging Trends Reshaping Recruitment Analytics
Recruitment is experiencing dramatic changes as new technologies offer better ways to find and evaluate talent. Analytics in recruitment is giving hiring teams powerful new capabilities to make smarter, data-driven decisions throughout the hiring process.
The Rise of Artificial Intelligence (AI)
AI is already transforming how companies recruit and hire. AI tools can now handle time-consuming tasks like screening resumes, scheduling interviews, and evaluating candidate skills. This allows recruiters to focus on building relationships with candidates and deeply understanding role requirements. AI systems can process thousands of applications quickly while reducing potential bias by focusing strictly on skills and experience.
Machine Learning's Impact on Predictive Hiring
Machine learning takes recruiting analytics further by identifying patterns that predict future outcomes. Companies can anticipate hiring needs, proactively source candidates, and identify which applicants are most likely to succeed in specific roles. By analyzing data from past hires, machine learning helps find candidates with traits similar to top performers, improving retention and reducing costly mis-hires.
Advanced Analytics for a Deeper Understanding
Modern analytics tools provide detailed insights into every stage of recruiting. Cohort analysis and predictive modeling help identify bottlenecks, optimize sourcing channels, and enhance candidate experience. This enables data-driven decisions across the entire process. For example, analytics can show which job boards deliver the best candidates for different positions, allowing smarter allocation of recruitment budgets.
Preparing for the Future of Recruitment Analytics
Success in modern recruiting requires embracing these new capabilities while carefully evaluating which tools best fit your needs and existing systems. Investing in training helps ensure recruiters can effectively use analytics tools and interpret the data they provide. Taking a strategic approach to analytics in recruitment will be key for attracting and keeping top talent.
Looking to improve your hiring process with AI and advanced analytics? Recrew offers 99.9% accurate resume parsing that automates data extraction and works seamlessly with your applicant tracking system.